Ultimate Guide to SaaS Auto Scaling
Learn about auto-scaling methods and strategies for SaaS applications, including cloud setups and future trends to optimize performance and costs.
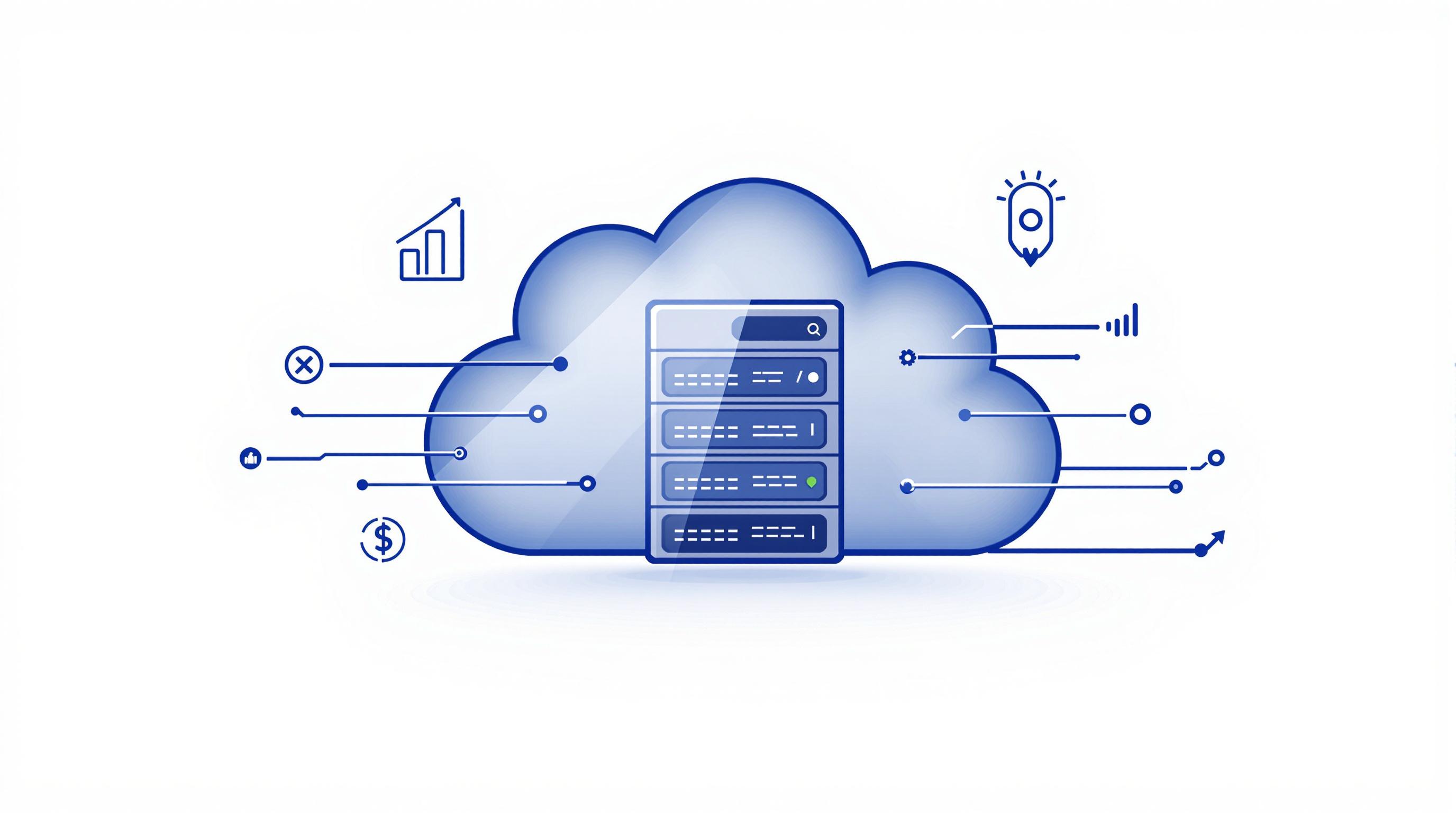
Auto-scaling ensures SaaS applications perform efficiently by automatically adjusting resources to match demand. Whether you’re managing sudden traffic spikes or planning for predictable growth, this guide covers everything you need to know about scaling methods, cloud platform setups, and future trends.
Key Takeaways:
-
Scaling Methods:
- Horizontal: Add more servers for stateless apps but requires managing network complexity.
- Vertical: Upgrade server resources, ideal for monolithic apps but has hardware limits.
-
Response Types:
- Real-Time: Adjust resources instantly based on performance metrics.
- Forecast-Based: Use historical data to predict and prepare for demand.
-
Cloud Platforms:
- AWS, Azure, and Google Cloud offer tools for auto-scaling, each suited for specific needs like microservices, .NET apps, or container-based workloads.
-
Future Trends: AI-driven scaling, multi-cloud strategies, and managing stateful applications are shaping the next generation of auto-scaling.
Quick Comparison:
Scaling Type | Best For | Limitations |
---|---|---|
Horizontal | Stateless apps | Network complexity, consistency |
Vertical | Monolithic apps | Hardware limits, downtime |
Cloud Platform | Key Features | Best For |
---|---|---|
AWS | Predictive scaling, integrations | Complex microservices |
Azure | Advanced monitoring, .NET focus | Windows workloads |
Google Cloud | Fast scaling, container support | Container-based applications |
Auto-scaling is essential for balancing performance and cost. Start with clear scaling goals, monitor performance metrics, and refine strategies using tools like Scale to Zero AWS to simplify implementation.
AWS Auto Scaling Deep Dive
Auto-Scaling Methods
Auto-scaling methods help manage traffic spikes by balancing system performance and cost.
Scaling Types: Horizontal and Vertical
There are two main ways to scale your system to handle increased load:
- Horizontal Scaling (Scaling Out): This involves adding more servers or instances to share the workload. It’s ideal for stateless applications and microservices architectures, as it allows infrastructure to grow with demand. However, it can introduce challenges like network complexity and maintaining data consistency.
- Vertical Scaling (Scaling Up): This method boosts the resources (CPU, RAM, storage) of an existing server. While easier to implement, it has hardware limits and may require downtime for upgrades.
Scaling Type | Best Use Cases | Key Limitations |
---|---|---|
Horizontal | Stateless apps, Microservices | Complex networks, Data consistency issues |
Vertical | Monolithic apps, Database servers | Hardware caps, Possible downtime |
The choice between these methods depends on factors like your application’s architecture and how predictable your workload is.
Response Types: Real-Time vs. Forecast
Auto-scaling can respond to demand in two main ways:
- Real-Time Response: This adjusts resources based on current performance metrics (like CPU or memory usage). It’s great for handling sudden, unpredictable workload spikes.
- Forecast-Based Response: This relies on historical data and predictive analytics to prepare for future demand. It’s a good fit for scenarios with predictable traffic patterns, like seasonal surges or regular business-hour peaks.
The response type you choose shapes how scaling is implemented.
Scaling Approaches
Auto-scaling can be triggered using various strategies:
- Target Tracking: Automatically adjusts resources to maintain specific performance goals, such as keeping CPU usage within a set range.
- Step Scaling: Adds or removes resources in fixed amounts when thresholds are reached, offering a more gradual adjustment.
- Scheduled Scaling: Allocates resources based on expected demand, making it ideal for recurring traffic patterns, like daily or weekly peaks.
- Metric-Based Scaling: Uses custom metrics, such as queue lengths or response times, to decide when to scale.
For SaaS applications that need flexible and efficient scaling, starting with a well-structured SaaS boilerplate can save time and effort. Platforms like Best SaaS Boilerplates offer starter kits with built-in features like authentication, multi-tenancy, and database management, simplifying the integration of auto-scaling strategies while keeping costs and performance in check.
Cloud Platform Setup Guide
Setting up auto-scaling on major cloud platforms can help balance performance and cost. Below are step-by-step instructions tailored for AWS, Azure, and Google Cloud to ensure a consistent approach to auto-scaling.
AWS Auto Scaling Setup
Steps to configure AWS Auto Scaling:
- Launch Template: Specify the instance details, including AMI, instance type, storage, security groups, and IAM roles.
- Auto Scaling Group Settings:
- Minimum size: 2 instances
- Maximum size: Based on peak load estimates
- Desired capacity: Baseline instance count
- Availability Zones: At least 2 for redundancy
- Scaling Policy: Use the following configuration to handle demand surges effectively:
{
"TargetValue": 70.0,
"PredefinedMetricSpecification": {
"PredefinedMetricType": "ASGAverageCPUUtilization"
}
}
This setup ensures your SaaS application adjusts dynamically to unexpected traffic spikes.
Azure Autoscale Setup
Steps to configure Azure Autoscale:
- Scale Set:
- Assign to a resource group
- Define an instance template
- Configure network settings
- Integrate with a load balancer
- Set Scale Rules: Apply the following rule to trigger scaling based on CPU usage:
{
"metricTrigger": {
"metricName": "Percentage CPU",
"metricNamespace": "Microsoft.Compute/virtualMachineScaleSets",
"threshold": 75,
"timeGrain": "PT1M",
"timeWindow": "PT5M"
}
}
- Monitor Integration:
- Track CPU usage
- Observe memory pressure
- Measure network throughput
- Include custom metrics for additional insights
These monitoring parameters help maintain system stability and performance.
Google Cloud Autoscaler Setup
Steps to configure Google Cloud Autoscaler:
- Instance Template:
- Define machine type
- Set up boot disk configuration
- Configure network settings
- Add startup scripts if needed
- Managed Instance Group:
- Choose regional or zonal deployment
- Select the instance template
- Configure named ports
- Set health check parameters
- Autoscaling Policies: Use the following example to manage scaling:
{
"cpuUtilization": {
"utilizationTarget": 0.6
},
"coolDownPeriodSec": 60,
"maxNumReplicas": 10,
"minNumReplicas": 2
}
Comparison of Key Features
Platform | Key Features | Best For |
---|---|---|
AWS | Predictive scaling, Multi-service integration | Complex microservices architectures |
Azure | Advanced monitoring, Custom metrics | .NET applications, Windows workloads |
Google Cloud | Easy setup, Fast scaling | Container-based applications |
When implementing auto-scaling, start with conservative thresholds and adjust them gradually based on real usage data and performance trends. This approach ensures efficient scaling without over-provisioning resources.
Auto-Scaling Setup Tips
Resource Planning
Start by understanding your application’s baseline needs and growth trends. Use multiple metrics - like CPU, memory, network usage, and application-specific data - to set scaling triggers. Relying on a mix of these indicators helps you scale more accurately and allocate resources effectively. After setting up, keep a close eye on performance to ensure your configurations are working as expected.
Performance Tracking
Keep tabs on your system’s performance to make sure auto-scaling is doing its job. Use observability tools to monitor response times, resource usage, and how long scaling actions take. Reviewing these patterns will help you fine-tune your scaling setup for better efficiency.
Current Issues and Future Direction
Auto-scaling strategies are evolving as new challenges and technologies reshape the landscape, building on existing methods and practices.
Scaling with State
Scaling stateful applications comes with its own set of hurdles. Traditional session management often struggles in distributed systems, leading to issues with session persistence. Modern approaches address this with distributed caching and smarter state management. For instance, Kubernetes StatefulSets provide built-in tools for maintaining pod identity and stable storage, simplifying the scaling of stateful workloads. Here are some key strategies for managing state:
- Using distributed session storage solutions like Redis or Memcached
- Employing event-driven architectures to keep state synchronized
- Implementing database sharding to enable horizontal scaling
AI and Serverless Scaling
AI is changing the game for auto-scaling by predicting workloads and fine-tuning resource allocation. Many serverless platforms now feature machine learning capabilities to forecast workload patterns, tackling challenges like cold starts. These platforms are also better equipped to handle complex workloads, running long processes and keeping instances warm when needed. Key advancements include:
- AI-driven capacity planning
- Automated resource optimization
- Real-time workload forecasting
- Resource allocation based on business priorities
Multi-Cloud Scaling
Scaling across multiple cloud providers adds complexity but also offers flexibility and resilience. Enterprises increasingly rely on multi-cloud strategies, making advanced orchestration essential. Cross-cloud tools now enable unified scaling policies, ensuring seamless performance across platforms. These tools help organizations:
- Set consistent scaling policies across different providers
- Use global load balancing for efficient traffic distribution
- Optimize costs by intelligently distributing workloads
- Ensure high availability through geographic redundancy
Solutions like Terraform Cloud and Crossplane make it possible to manage auto-scaling uniformly across AWS, Azure, and Google Cloud. These tools extend traditional scaling methods, pushing the limits of what modern SaaS platforms can achieve.
SaaS Boilerplates for Scaling
Boost your auto-scaling strategies with SaaS boilerplates that simplify infrastructure setup and ensure scalability.
Boilerplate Basics
SaaS boilerplates speed up the development of scalable applications. They come with essential features like authentication, multi-tenancy, database management, monitoring, and logging - all designed to support efficient scaling on cloud and serverless platforms. These tools incorporate best practices for handling load, managing state, and caching, creating a solid foundation for your app to grow in line with your auto-scaling strategies.
Key Features to Look For
When picking a boilerplate, prioritize features that align with your scaling goals. Platforms like Best SaaS Boilerplates offer curated directories with filters for cloud provider integration and specific capabilities.
Here’s a quick comparison of essential features in popular boilerplates:
Feature | Purpose | Scaling Benefit |
---|---|---|
Multi-tenancy Support | Keeps customer data isolated | Allows separate scaling for each tenant |
Serverless Architecture | Simplifies infrastructure management | Automatically adjusts to demand |
Distributed Caching | Speeds up data access | Reduces database load during scaling |
Load Balancing | Evenly spreads traffic | Avoids bottlenecks |
A great example of success comes from Makerkit. In March 2023, Buomprisco LTD used a Next.js and Remix boilerplate to launch three SaaS products in just six months. Thanks to pre-built components, development time was cut by 40%, while scaling remained smooth and efficient.
”A SaaS boilerplate where you can choose your favorite tech stack combos - Next.js, Remix, Firebase, Supabase - to build unlimited SaaS products in record time. So good it feels like cheating!”
– Giancarlo Buomprisco, Makerkit
Advanced search tools on these platforms help developers find boilerplates tailored to their needs, whether they require integration with AWS, Azure, or Google Cloud. As Jonathan Wilke from Supastarter puts it, “Save endless hours of development time and focus on what’s important for your customers. Get everything you need to launch your SaaS like auth, payments, i18n, mails and more”.
Choosing the right boilerplate involves assessing factors like compatibility with your infrastructure, monitoring tools, distributed state management, and database scalability. Many boilerplates also include CI/CD pipelines and support tools like Kubernetes, making horizontal scaling easier and more efficient.
Summary
Main Points
Auto-scaling in SaaS requires careful planning to ensure both performance and cost-efficiency. Here’s a quick look at the key elements:
Component | Key Considerations | Impact |
---|---|---|
Scaling Methods | Horizontal vs. Vertical | Determines how flexible resource allocation can be |
Response Types | Real-time vs. forecast-based | Affects how quickly the system reacts to load changes |
Platform Integration | AWS, Azure, Google Cloud | Impacts available tools and associated costs |
Resource Management | Performance tracking and cost control | Ensures efficient use of resources |
These elements are crucial for building a reliable auto-scaling strategy. To succeed, focus on three key areas:
- Infrastructure Planning: Build an infrastructure that supports both horizontal and vertical scaling.
- Monitoring and Metrics: Keep an eye on important KPIs like CPU usage, memory, response times, and queue lengths.
- Cost Optimization: Use a mix of schedule-based, event-driven, and predictive scaling rules to manage costs effectively.
Modern SaaS boilerplates simplify auto-scaling by offering pre-built components tailored for various use cases. Remember, auto-scaling isn’t just about managing higher traffic - it’s about keeping performance high while controlling expenses. Whether you’re using AWS, Azure, or Google Cloud, base your scaling policies on real-world usage data for better results.
Recommended SaaS Boilerplates
Highly recommended SaaS boilerplates you can use to build and scale your startup: